1. Optical image processing and AI: an unbeatable combination
Artificial intelligence (AI) is no longer just a vision of the future. It is already a transformative reality in the industry. Its use is fundamentally changing production, management and maintenance in particular. AI not only enables an increase in efficiency, but also brings along previously unimaginable adaptability and precision, especially in the manual manufacturing industry
But what exactly is AI, and why is it so important for modern industrial processes and their quality assurance?
AI refers to systems or machines that are capable of performing tasks that traditionally require human cognitive thinking. This ranges from recognizing complex patterns to intelligent image processing (using machine learning). Based on AI, systems are able to make decisions independently and optimize processes.
And why is it so important for modern industrial processes and their quality assurance? Find out in this blog post.
SK2Go for easy license management
Unlimited use and recipe creation with the new USB dongle. Versatile use in production. Read more about "SK2Go for easy license management"...
AI in industry: The future of quality assurance through optical precision
Efficient quality control at Prettl Electronics GmbH with worker assistance system for THT assembly. Fully digital system interlinking. Read more about "AI in industry: The future of quality assurance through optical precision"...
Tier 1 Suppliers ensure Quality with Worker Assistance Systems
Tier 1 supplier chooses worker assistance system for automatic quality assurance of end-of-line testing. Read more about "Tier 1 Suppliers ensure Quality with Worker Assistance Systems"...
2. The rise of AI in Industry 4.0
The mid-20th century saw the start of AI as a discipline in computer science with the aim of simulating human intelligence.
Today, tremendous progress has been made and it is hard to imagine everyday life without the use of intelligent assistance. This has only been made possible by improved computer performance and the accessibility of data, as well as the programming of increasingly advanced algorithms.
The increasing digitalization and interlinking of production processes, known as Industry 4.0 and the Internet of Things, has brought about a significant change in the industry. Since then, the productive potential has been growing daily.
Today, AI in industry can not only automate and optimize processes, but also enable unprecedented flexibility and individualization of production. It allows machines to analyze data in real time in order to make decisions, maximize production efficiency, avoid errors or plan maintenance with foresight. This makes production processes more efficient, more adaptive, safer, and consequently more economical.
The role of artificial intelligence in Industry 4.0 is therefore fundamental: it is pushing forward the development of smart factories in which machines and systems communicate and cooperate independently. With the help of AI-based tools, companies can adapt their production flexibly to process changes, use resources more efficiently and ensure higher product quality - especially in the area of manual assembly.
In short, artificial intelligence is the engine that is leading the industry into a new era of innovation, efficiency, and flexibility.
3. Overview: Areas of application for AI in industry
In the industrial sector, artificial intelligence is used along the entire value chain, from production to marketing. Companies use it to increase their productivity and develop innovative solutions:
- Quality assurance: In THT assembly, artificial intelligence is used for optical real-time monitoring. All kinds of industries use specialized AI algorithms to detect components that are difficult to distinguish in a matter of seconds and thus make their variant complexity easier to handle. Algorithms automatically and reliably detect differences, irregularities, or defects that humans cannot always consciously perceive due to various influencing factors. Errors in (printed circuit board) production, for example, are detected at an early stage with the help of optical inspection, which leads to a reduction in rejects and consequently, scrap costs. This increases the profitability of production processes.
- Logistics and supply chain management: In the logistics industry, for example, service providers use predictive analytics or intelligent route planning to optimize delivery times. AI systems analyze historical delivery data and traffic conditions to predict the most efficient routes and delivery times, which leads to a significant increase in efficiency in the supply network.
- Maintenance and anomaly detection: Large industrial companies use AI-based predictive maintenance algorithms that continuously analyze data from sensors to detect potential machine failures or maintenance needs at an early stage. This early detection enables efficient planning of maintenance work and minimizes unplanned downtime, which can save significant costs.
- Assembly: Automotive manufacturers are implementing AI-based robots to optimize assembly processes and increase productivity. They are able to carry out complex tasks, such as assembling small components with maximum precision. Using sensors or data analysis, they understand their environment. Based on this, they make decisions and can react more flexibly to changes in production. AI robots learn and improve over time by collecting and schematically analyzing the collected data.
4. Using AI for optical quality assurance
In manual production, where precision and accuracy are of the utmost importance, employees face numerous challenges every day. Ensuring consistent quality is particularly important - despite the large number of variants and the complexity of the features to be inspected. Low volume high mix is a term that is increasingly shaping manual production.
In the course of everyday production, components are often mixed up. Optical quality assurance, traditionally carried out by the trained human eye, often reaches its limits here.
AI-based image processing software, on the other hand, for instance in a digital assistance system, can detect even the smallest differences using real-time image acquisition. It can access thousands of taught-in features in a matter of seconds and compare them with image databases. This allows the system to check whether the necessary parts are present and whether the positioning or polarity is correct. Once the correct part has been positioned correctly, the process can continue. The error-prone 4-eye check is no longer necessary, and the digitized process becomes safer and more efficient.
In order to obtain more precise results from AI and to be able to react to a greater number of variants, the underlying neural networks must be continuously trained. So-called annotation plays a major role in machine learning here. (Image) data is provided with relevant information so that it can learn patterns and correlations.
For annotation, labels are added to thousands of pieces of data to show the network which patterns it should recognize and search for.
A number of AI systems are able to recognize and differentiate between shapes, even if they differ only minimally from one another. In manual assembly, the clear advantage is that with a high variety of variants, the workload on humans is reduced and errors caused by mix-ups are avoided.
- Machine learning: Machine learning refers to algorithms and techniques that enable computers to learn from data, recognize patterns and automatically gain insights without receiving explicit instructions.
- Neural networks: Neural networks are a specific machine learning technique inspired by the biological structure of the brain. They consist of interconnected artificial neurons that are arranged in layers and can process information.
Text can also be recognized and processed using Deep OCR technology (image to text). With the help of advanced image processing algorithms, the AI is able to identify and extract text from images in real time, even from different perspectives. This is particularly advantageous when it comes to the labeling, identification and traceability of products. Important information on the components or packaging is automatically captured and processed.
In order to obtain more precise results from AI and to be able to react to a greater number of variants, the underlying neural networks must be continuously trained. So-called annotation plays a major role in machine learning here. (Image) data is provided with relevant information so that it can learn patterns and correlations.
For annotation, labels are added to thousands of pieces of data to show the network which patterns it should recognize and search for.
Employees can concentrate on more complex tasks while the AI takes over the routine but error-prone inspection work (see image). The use of AI in optical quality assurance for manual production minimizes sources of error, guarantees traceability and increases product quality. The workload for employees is also significantly reduced.
5. Conclusion
The rapid development of artificial intelligence and its ubiquitous use indicate by no means that its potential in industry has been fully exhausted - especially in the field of optical quality control.
Artificial intelligence is of great importance as it offers new approaches to the complex challenges of modern industry.
Companies that are willing to embrace and invest in these technologies will not only increase their efficiency and productivity, but also open up new business models and opportunities.
The future of industry will be increasingly shaped by smart technologies and AI. Those who drive and strategically deploy these technologies will be instrumental in shaping the industrial landscape of tomorrow and staying ahead of the competition.
Ready to take off with Smart Klaus
Customer Success Stories
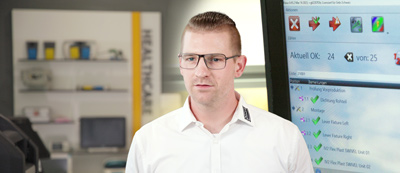
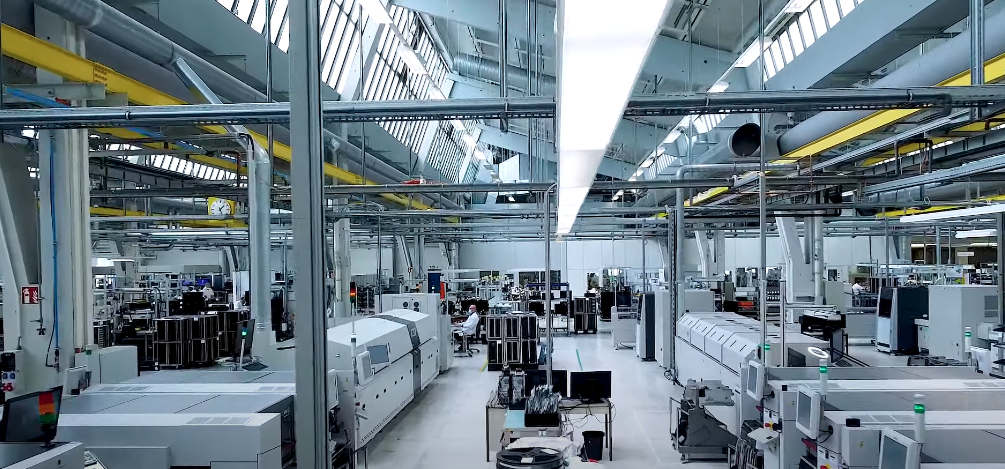
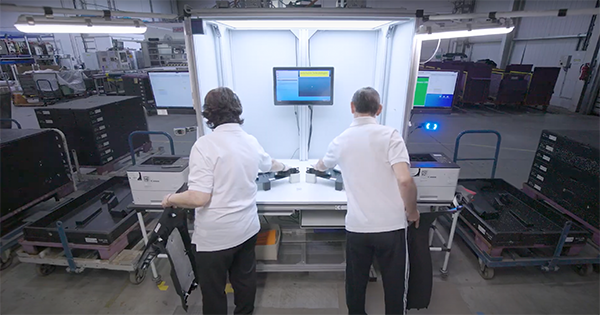
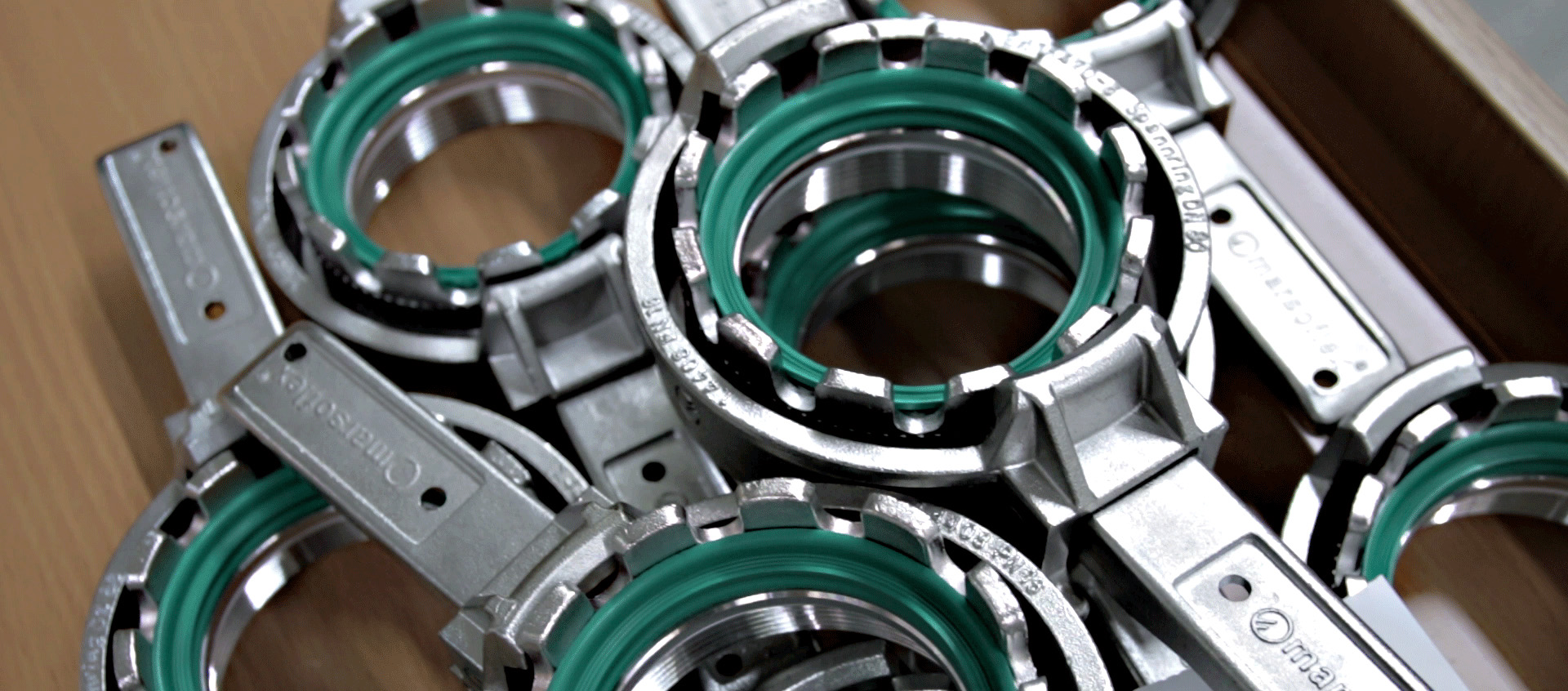